Artificial intelligence in medicine: how AI accelerates clinical trials and drives innovation
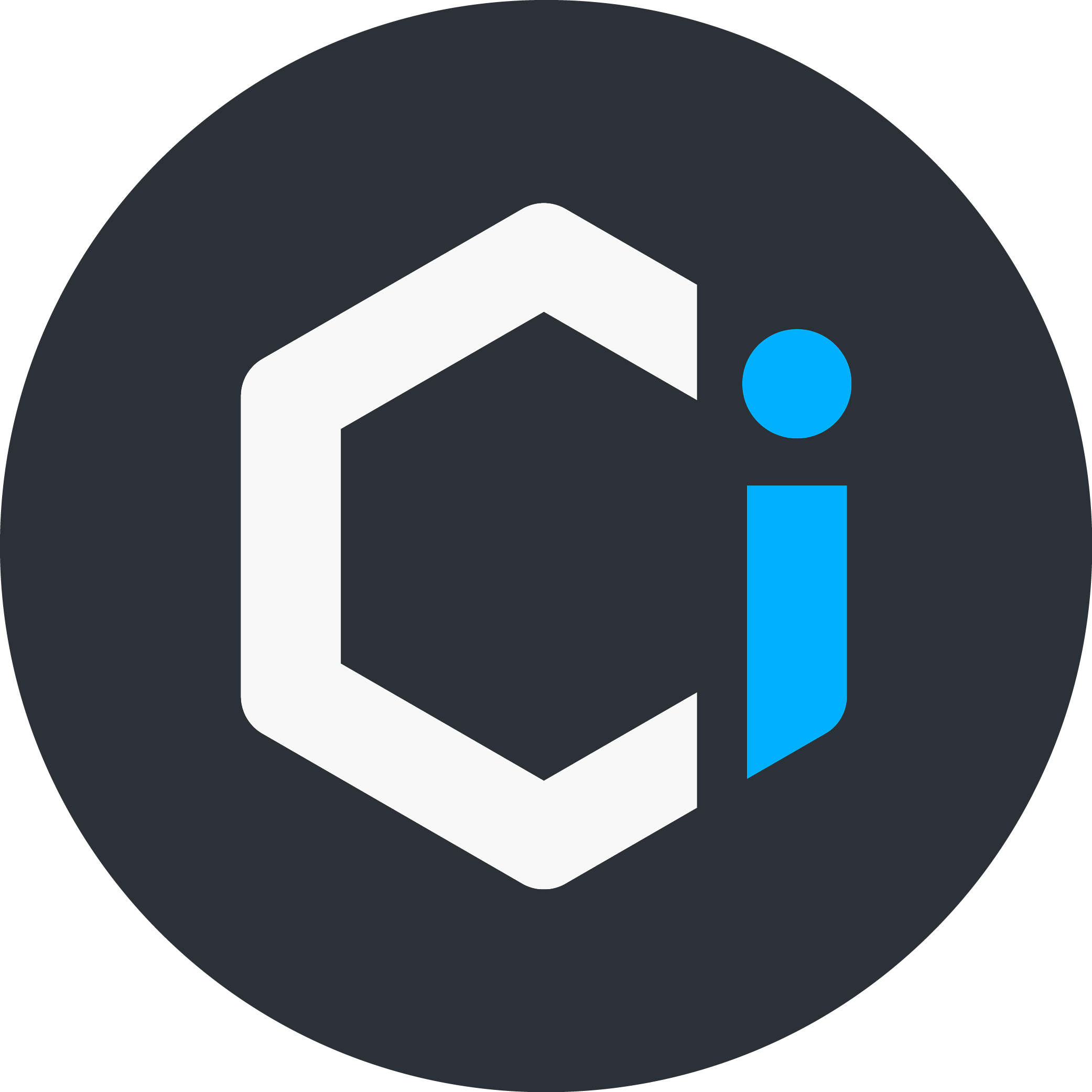
Authored by
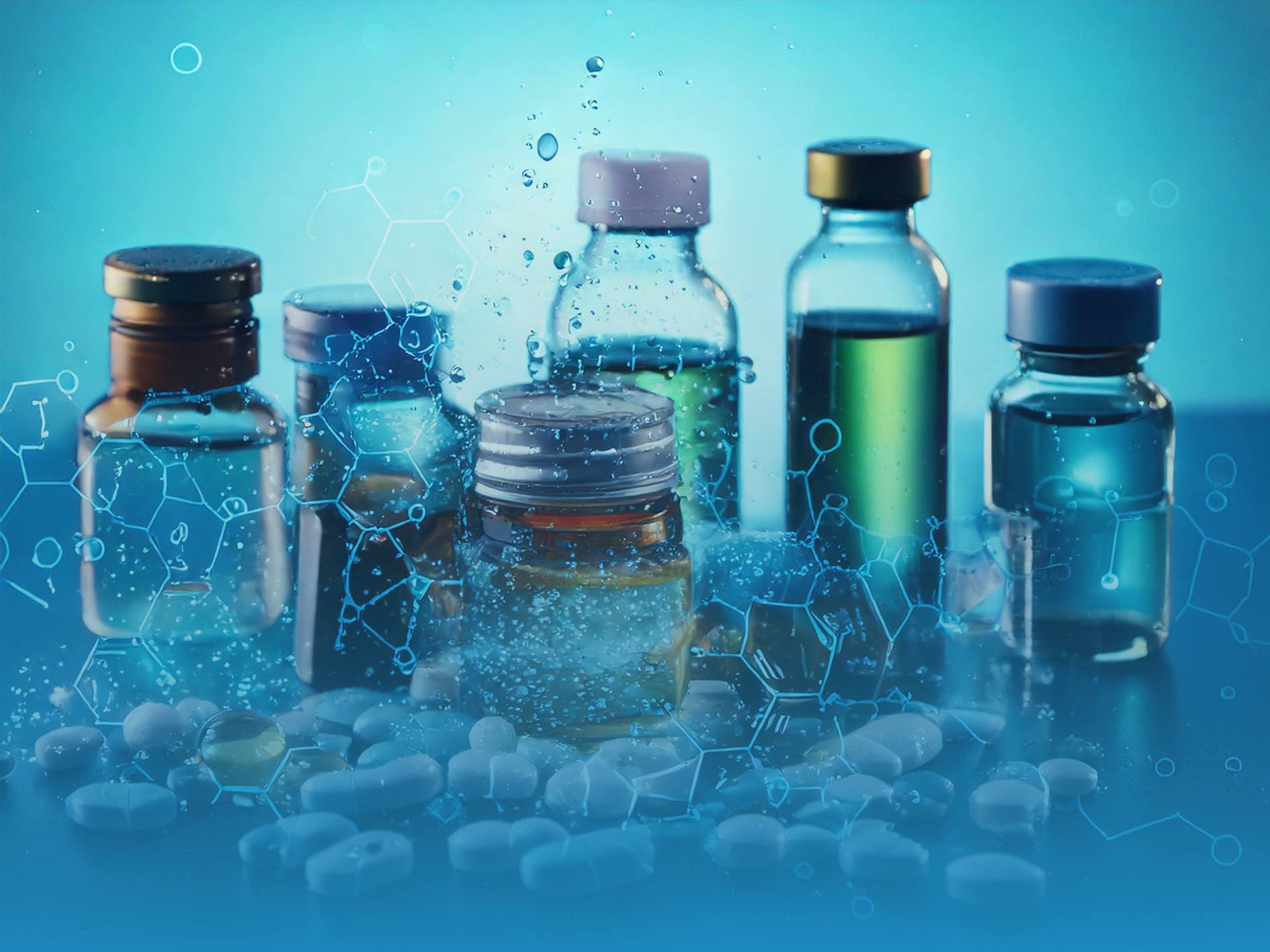
October 9, 2024
Clinical research is facing a paradigm shift: the use of data and artificial intelligence (AI) is opening up new opportunities for medicine to work faster and more precisely. AI not only helps to make clinical trials more efficient, but also to increase the safety and accuracy of research results. In a world where new therapies often take years to develop, data-driven medicine can pave the way for rapid and future-proof innovation. In this article, you will learn how AI optimizes clinical trials, what challenges arise and what concrete strategies companies can use to take advantage of these new opportunities.
Data-driven medicine and AI: an overview of current trends
In modern medicine, the integration of data and AI is no longer a futuristic concept. With increasingly powerful computers and the availability of large amounts of data, clinical research is currently undergoing a revolution. Traditional study processes that once took years are now being accelerated with the help of algorithms. These technologies allow researchers to recognize patterns from countless patient data, validate hypotheses and manage clinical trials in a more targeted manner.
A key aspect of data-driven medicine is the automation and optimization of study processes. Traditionally, researchers had to analyze data manually, which was time-consuming and error-prone. Today, however, AI-supported systems make it possible to analyze large amounts of data efficiently and uncover hidden correlations. The potential of AI is particularly evident in personalized medicine, where therapies are tailored to specific genetic or health profiles. Data-driven medicine makes it possible to design results more precisely and minimize side effects, which increases both the safety and effectiveness of treatments.
The role of AI in clinical trials goes beyond pure data analysis. Machine learning and deep learning make it possible to make well-founded predictions from data and identify any risks at an early stage. This enables targeted adjustments to the course of the trial and increases the probability of success. Data-driven medicine is therefore not just a trend, but is increasingly becoming an indispensable tool in clinical research.
Opportunities and challenges of data-driven medicine
Despite its enormous benefits, the integration of AI into clinical trials brings both opportunities and challenges. Here are some key aspects that companies and research institutions should consider:
1. data quality and accessibility:
For AI models to deliver reliable results, the underlying data must be complete, accurate and up-to-date. However, one of the biggest challenges is to consolidate data from different sources and ensure that it is standardized and accessible. Patient data is often fragmented and stored in different systems, making integrated analysis difficult.
2. data protection and ethical issues:
The handling of patient data is a sensitive topic, especially in medicine. Data protection directives such as the GDPR in Europe set strict requirements for the use and processing of personal data. For the successful implementation of AI in clinical trials, companies and research institutions must ensure that they comply with legal requirements and respect ethical standards.
3. adaptation of study methods:
The use of AI often requires an adaptation of traditional study methods. While randomized control trials were previously the gold standard, AI-supported methods offer the opportunity to design studies adaptively. However, it is important to continuously validate the AI models and ensure that the results remain clinically relevant.
4. costs and resources:
The implementation of AI is not free and requires specialized resources and expertise. In addition to the initial investment in the technology, companies need to invest in qualified personnel and infrastructure to ensure long-term success.
5. skills shortage:
The development and management of AI in clinical research requires specialized knowledge in data science and medicine. Finding qualified specialists can be time-consuming and slow down implementation. Collaboration with specialized service providers can offer a solution here.
6. compliance and regulatory requirements:
To ensure the safety and efficacy of medical products, clinical trials are subject to strict regulatory requirements. Compliance with these standards is essential, but the implementation of AI can make regulatory processes more complex. It is therefore crucial to choose AI systems that can be seamlessly integrated into existing compliance structures.
Strategies for the successful implementation of AI in clinical trials
The successful use of AI in clinical trials requires a well thought-out approach and concrete strategies. Companies and research institutions wishing to take advantage of this technology should consider the following tips:
1. building a stable data infrastructure
A solid data infrastructure is the backbone of any AI application. Companies should focus on consolidating their data sources and ensure that the data is easily accessible, standardized and of high quality. The introduction of a central platform that brings together different data streams can be crucial here.
2. use of anonymized data
To protect patient data, it is important that data is anonymized before it is used in AI models. This ensures that sensitive information remains protected and that data protection requirements are met. Anonymized data enables flexible use for research without compromising patient privacy.
3. introduction of real-time analyses
Real-time analyses make it possible to continuously monitor study processes and react flexibly to new data. AI-supported analyses enable companies to identify any deviations at an early stage and adjust the course of the study if necessary. This increases the efficiency of studies while ensuring the safety of participants.
4. validation of AI models
In order for AI-supported study methods to remain scientifically recognized, continuous validation of the models used is necessary. This ensures that the results are reliable and clinically relevant. Regular checks and updates of the AI algorithms are essential to ensure the quality of the studies.
5. implementation of predictive analytics for risk analysis
With the help of predictive analytics, companies can better assess and identify risks in clinical trials at an early stage. Predictive risk analysis makes it possible to avoid potential complications and make study processes more efficient. Predictive analytics is particularly useful for identifying side effects and adapting treatment methods.
6. cooperation with specialized partners
As the development and implementation of AI is complex, partnerships with specialized providers can be beneficial. External partners offer not only the necessary technologies, but also the expertise to ensure that AI integration runs smoothly and regulatory requirements are met.
7. employee training
The successful implementation of AI requires a certain level of expertise among employees. Through targeted training, companies can ensure that their team makes optimal use of the new technologies and feels up to the challenges. Investing in staff training pays off in the long term and helps to increase the efficiency and accuracy of study processes.
How CorpIn supports clinical research with AI solutions
CorpIn offers customized solutions to support companies in the integration of AI into clinical trials. Our experts develop strategies that improve the efficiency and quality of study processes without neglecting strict compliance and data protection requirements.
One example is our initial analysis of the data and system infrastructure. Before AI solutions are implemented, we analyse the existing data landscape and identify weaknesses and optimization potential. The aim is to create a solid database on which the AI models can work reliably. This initial analysis is crucial in order to consolidate data sources and lay the foundation for data-driven studies.
Another example is the automated analysis of clinical studies. CorpIn implements AI models that can efficiently analyze large amounts of data and thus shorten the study time. By using machine learning, patterns and correlations in the data can be identified that are valuable for further study development.
An additional focus is on predictive analytics for risk analysis. Predictive analysis models enable companies to identify potential risks and side effects at an early stage and take targeted measures. Our solutions help to make studies safer and more effective and maximize efficiency.
Finally, CorpIn ensures that all AI-powered solutions meet the highest data protection standards. With our expertise in compliance and security, we help companies meet regulatory requirements while taking full advantage of the benefits of data-driven medicine.
Conclusion
Data-driven medicine has the potential to fundamentally change clinical research. By using AI, companies can accelerate clinical trials, reduce risks and improve the quality of their research. Despite the challenges, AI integration offers enormous opportunities for developing new therapies and improving patient safety.
CorpIn supports companies on their path to data-driven research - from data infrastructure to the automation of analyses and predictive analytics. Discover how our customized solutions increase the efficiency and quality of your study processes while meeting the highest data protection and compliance requirements.
The content of this article may have been improved with the help of artificial intelligence. Therefore, we cannot guarantee that all information is complete and error-free.