Efficient agriculture through AI: how machine learning increases crop yields
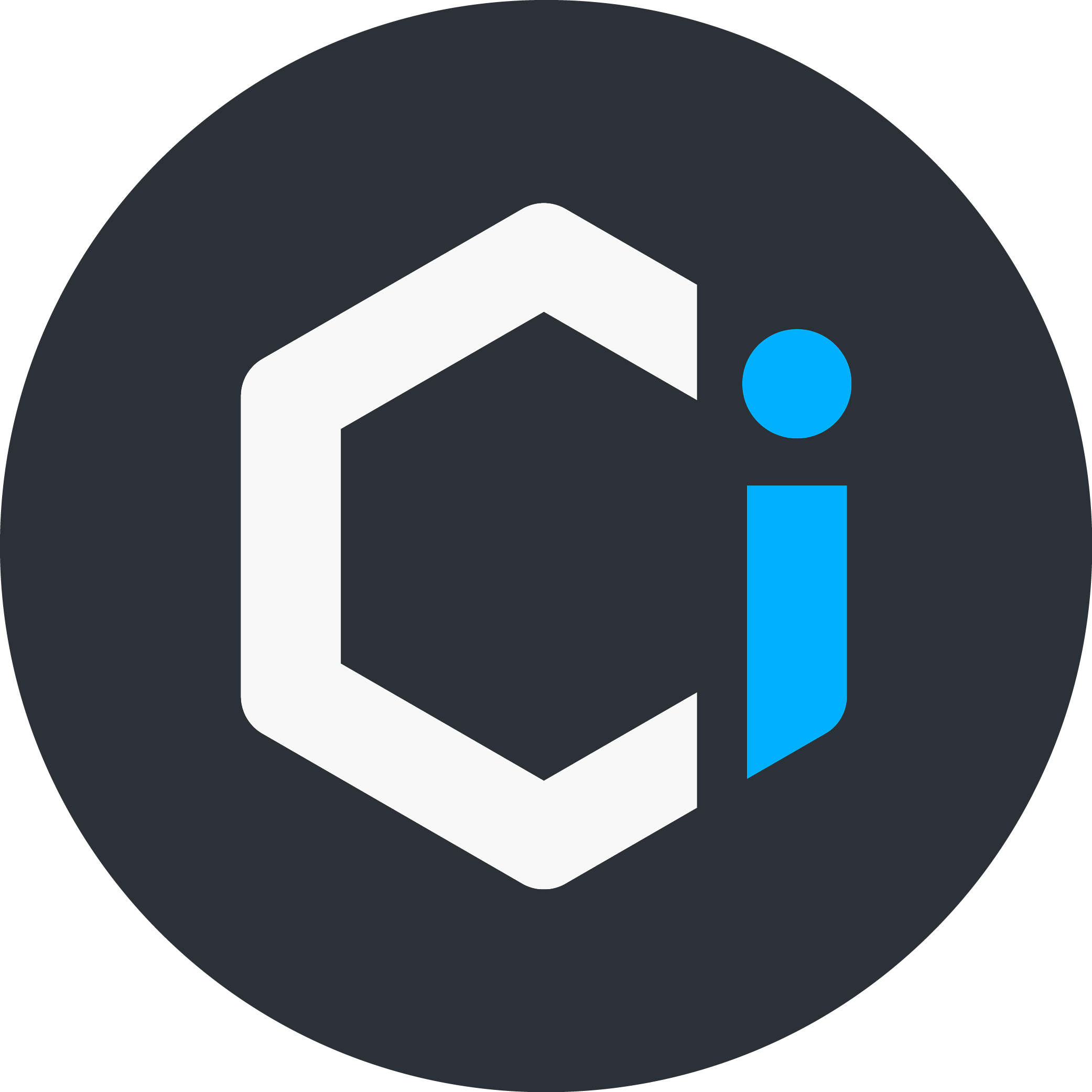
Authored by
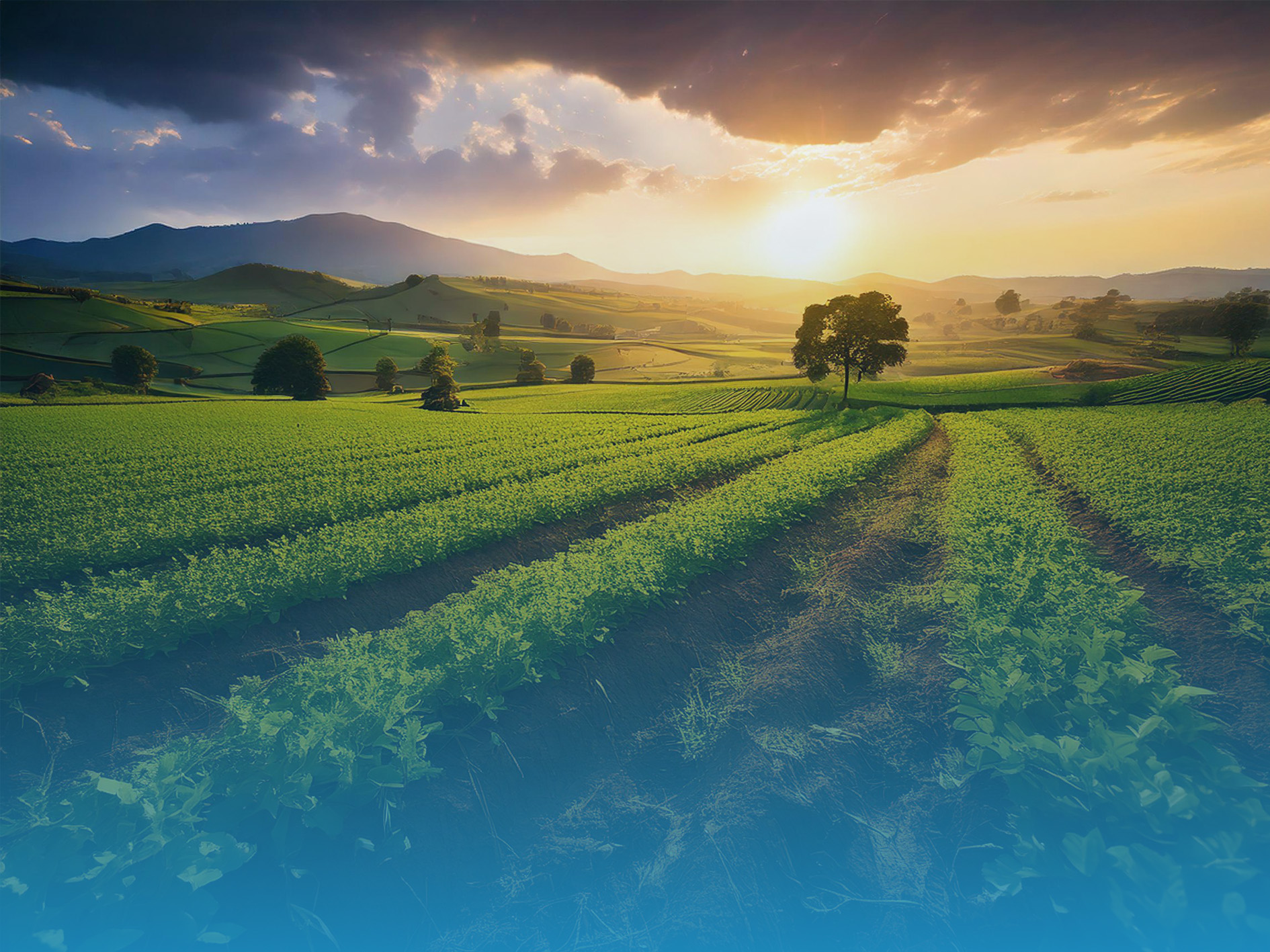
August 12, 2024
Agriculture is facing major challenges worldwide: Weather conditions are changing rapidly, resources such as water are becoming scarcer and the demand for food is constantly increasing. In this context, the use of artificial intelligence (AI) is becoming increasingly important. Machine learning enables farmers to plan harvests more precisely, use resources more efficiently and increase productivity. This article looks at how AI and machine learning are revolutionizing the agricultural sector and the concrete benefits for farmers and companies.
Overview: AI and machine learning in agriculture
Recent years have shown that AI-based solutions can significantly increase efficiency in agriculture. By integrating technologies such as drones, sensors and satellite images, large amounts of data are collected, which are precisely analyzed and evaluated thanks to machine learning. The result is a data-driven agriculture that is based on sound knowledge and can react quickly to environmental conditions.
Today, AI solutions enable precise analysis of soil quality, monitoring of plant growth and predictive detection of pests and diseases. These technologies help farmers to make more informed and faster decisions. Combined with advanced machinery such as autonomous tractors and harvesters, this creates a smart, efficient agriculture that meets the demands of the future.
The demand for such technologies is increasing: The market for AI in agriculture is growing worldwide and shows that awareness of increasing efficiency and sustainable production is growing. Machine learning in particular, a form of AI that continuously learns through pattern recognition and experience, is taking agriculture to a new level of optimization.
Challenges and opportunities in the use of AI in agriculture
Challenges
1. access to high-quality data:
Machine learning requires large amounts of high-quality data. However, this is not always easy to obtain. Small farms in particular often do not have the infrastructure or the financial means to install sensors and data collection devices. This creates a gap between large and small farms, which can have a long-term impact on competitiveness.
2. high investment costs:
The introduction of AI-based systems can be costly. The technologies are complex and implementation can require investment in new machinery and software solutions. For many companies, this is a financial challenge, especially in the initial phase when the return on investment is still uncertain.
3. data protection and security:
The use of technologies such as drones and sensors means that large amounts of data are collected and processed. This data is valuable and must therefore be securely protected against cyber attacks. Data protection guidelines must also be taken into account, as data security is a high priority for companies and customers.
Opportunities
1. increased yield and efficiency:
Yields can be significantly increased through more precise cultivation methods and better monitoring of plant growth. Machine learning helps farmers to use resources in a targeted manner and only irrigate or fertilize where necessary. This leads to cost savings and an increase in productivity.
2. sustainability and environmental protection:
The efficient use of resources such as water and fertilizers helps to reduce the ecological footprint. In addition, pesticides can be used in a more targeted manner, which protects the environment and improves soil quality in the long term. AI-based systems support farmers in making environmentally friendly decisions.
3. reduced workload:
Autonomous machines and AI-supported monitoring systems reduce the physical strain and workload of farmers. Routine tasks such as monitoring crops or analyzing soil samples can be automated, allowing farmers to focus on strategic decisions.
Practical solutions and strategies for the introduction of AI in agriculture
The introduction of AI in agriculture requires a structured approach and the use of suitable technologies. Companies and farmers can follow the following strategies to maximize the benefits of machine learning:
1. integration of sensor technology:
Sensors are the backbone of data-based agriculture. They provide information on soil moisture, nutrient content and weather conditions. Farmers can thus recognize when and where to water or fertilize, which makes the use of resources much more efficient.
2. use of drones for field monitoring:
Drones with high-resolution cameras enable visual monitoring of large fields. Regular flights allow irregularities in plant growth or pest infestations to be detected at an early stage. Data analysis is carried out using machine learning, which recognizes patterns in the images and makes recommendations for field management.
3. forecasting models for weather and harvest:
By analyzing historical weather data and current weather conditions, AI can create forecasts that help farmers determine the ideal time to harvest. This is particularly important in order to minimize weather-related risks and plan the harvest optimally.
4. precision agriculture for needs-based use of resources:
Precision agriculture uses machine learning to determine which plants need which care at which time. This applies to irrigation, fertilization and the use of pesticides, which are applied in a targeted and resource-saving manner. This reduces costs and increases crop yields.
5. partnerships and pilot projects:
Getting started with AI technologies can be challenging, especially for companies that have little experience with digital technologies. Cooperation with technology service providers or starting with a pilot project are ideal ways to gain initial experience and adapt the technology to specific needs.
How CorpIn supports agriculture with AI solutions
As an expert in data-driven process optimization and AI-supported strategies, CorpIn offers support for agricultural businesses that want to modernize and optimize their processes. Our approach is based on the individual analysis and integration of technologies that are specifically tailored to the requirements of agriculture.
Our services include consulting and implementation of sensor and drone technology that enables continuous monitoring of fields. In addition, we offer tailor-made solutions for data analysis and evaluation that provide farmers with valuable insights into their operational processes, allowing them to optimize the use of resources in a targeted manner.
At CorpIn, we understand that the success of AI solutions in agriculture depends on the quality of the data and the user-friendliness of the systems. Our experts help companies ensure data quality and develop customized solutions that are intuitive to use. Our goal is to increase agricultural productivity, optimize resource consumption and help farmers to operate sustainably and profitably.
Conclusion
The use of AI and machine learning in agriculture offers enormous opportunities, from increasing yields to conserving resources. Through the targeted analysis of soil data, the use of autonomous machines and the use of drones for field monitoring, farmers can make their processes more efficient and increase productivity. However, the introduction of AI also brings challenges such as high investment costs and data security requirements.
CorpIn offers companies the support they need to successfully put these technologies into practice and benefit from the advantages of data-driven agriculture in the long term. With the right strategy and an experienced partner at their side, agricultural businesses can take the step into the future and raise their efficiency and sustainability to a new level.
The content of this article may have been improved with the help of artificial intelligence. Therefore, we cannot guarantee that all information is complete and error-free.