Predictive maintenance: how predictive maintenance reduces downtime and saves costs
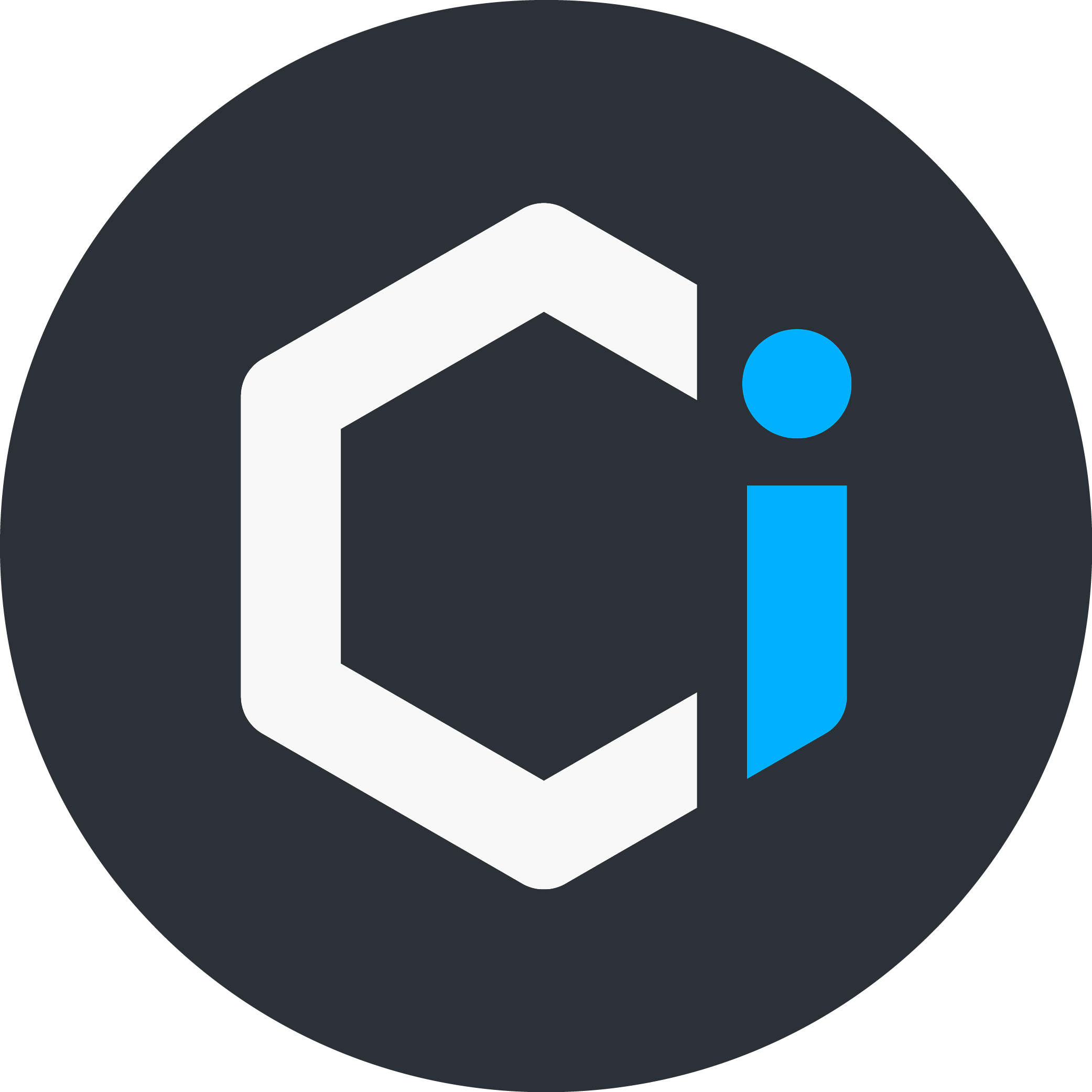
Authored by
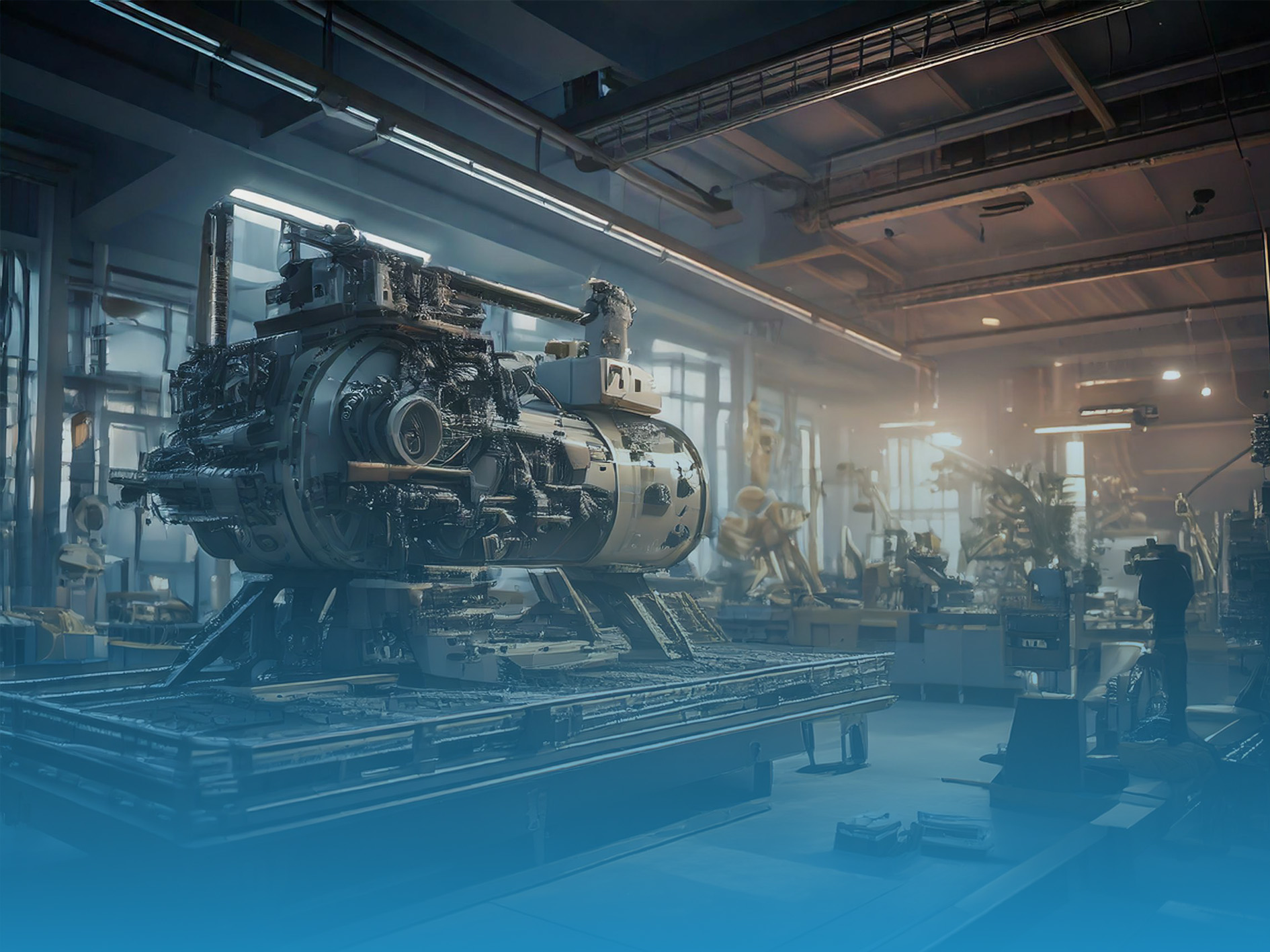
October 17, 2024
Unplanned downtime in production can cost companies dearly. Traditional maintenance is often not enough to repair machines in time and prevent production downtime. This is where predictive maintenance comes into play: predictive maintenance uses artificial intelligence (AI) and data analysis to identify problems before they arise. This approach enables predictive maintenance, which not only saves costs but also increases reliability and productivity. In this article, you will learn how predictive maintenance works, what opportunities and challenges it offers and how companies can benefit from this technology with targeted strategies.
Predictive maintenance: trends and developments
With the rapid pace of digitalization and technological progress, interest in predictive maintenance is increasing. Traditional, cyclical maintenance systems are often based on fixed time intervals or the empirical value of how long a machine runs without malfunctions. However, this model is inefficient as it intervenes either too early or too late, which inevitably leads to production downtime or unnecessary maintenance costs.
Today, sensors, the Internet of Things (IoT) and advanced data analytics tools offer new opportunities. By integrating sensors into machines, companies can access real-time data that measures everything from temperature and vibration to a system's performance. AI and machine learning can be used to identify patterns and deviations that indicate a potential problem. Predictive maintenance makes it possible to forecast impending failures and schedule maintenance work in good time.
The trend is no longer to view maintenance as a fixed cost, but as a strategic factor in production planning. Companies that implement predictive maintenance not only benefit from a reduction in downtimes, but also from optimized maintenance cycles, better production processes and increased efficiency.
Challenges and opportunities when introducing predictive maintenance
The introduction of predictive maintenance brings numerous benefits, but there are also challenges that need to be considered. A detailed look at the opportunities and hurdles helps companies to choose the right strategy.
1. data availability and quality:
Predictive maintenance is data-based - the availability and quality of the data are therefore crucial for success. One challenge is that many companies store data in different systems that are not linked to each other. A solution is therefore needed that combines data sources and ensures data quality.
2. implementation costs:
The introduction of predictive maintenance is an investment that requires initial costs for hardware, sensors, software and employee training. Companies must weigh up how to distribute the costs and where to prioritize them. However, the costs are amortized in the long term through savings and the increase in machine availability.
3. skills shortage:
The successful implementation and maintenance of predictive maintenance systems requires expertise in the fields of data science, mechanical engineering and IT. The lack of qualified personnel can slow down the process. The right mix of internal training and external expertise is therefore crucial.
4. data protection and cyber security:
As predictive maintenance is based on IoT and cloud solutions, data protection is an important issue. Companies must ensure that all data is processed and stored within a secure framework. Cybersecurity is particularly important to protect production from potential attacks.
5. optimization of the maintenance process:
The introduction of predictive maintenance often requires a realignment of existing maintenance processes. This means replacing existing processes, such as fixed maintenance intervals, with more flexible, data-driven maintenance approaches. This changeover can be a challenge, but offers the opportunity to make the entire maintenance process more efficient.
6. cultural changes and employee acceptance:
The introduction of new technologies often entails a change in corporate culture. Employees who are used to traditional maintenance methods have to adapt to the new system. Successful change management is essential in order to strengthen the workforce's trust in predictive maintenance.
Practical strategies for the successful implementation of predictive maintenance
Predictive maintenance can only be implemented successfully if companies take a strategic approach and actively tackle the challenges. The following strategies help to successfully and efficiently integrate predictive maintenance into existing processes:
1. development of a solid data strategy:
Before predictive maintenance can be implemented, a well thought-out data strategy must be developed. Companies should analyze their existing data sources and ensure that the data is consistent and of high quality. It is also helpful to implement a central data platform that enables all relevant information to be brought together.
2. use and process sensor data:
To monitor the condition of machines, companies should integrate sensors into their systems. These sensors collect real-time data, which is then analyzed by AI models. Temperature, vibration and power are some of the parameters that can be measured and analyzed to detect potential problems at an early stage.
3. choosing the right technology:
Predictive maintenance requires specialized software and hardware. Companies should carefully select the technology that best suits their requirements. Different platforms offer different possibilities - a solution that can be easily integrated into existing systems often has an advantage.
4. pilot projects and gradual implementation:
It is advisable not to implement predictive maintenance across the entire company at once, but to start with pilot projects. This allows the company to gain experience and test the technology within a controlled framework. This gradual introduction makes it possible to make optimizations before the system is scaled up.
5. employee training:
Employees must be trained in the new technologies in order to use the systems effectively. Regular training and workshops are helpful to promote know-how within the team and increase acceptance of predictive maintenance.
6. partnerships with specialized providers:
The implementation and maintenance of predictive maintenance systems can be a challenge. Working with external partners who specialize in the development and implementation of such systems can help to make the best use of knowledge and resources.
7. establishment of cyber security measures:
Predictive maintenance also entails security risks through the use of the Internet of Things. Companies should implement cybersecurity protocols and ensure that their systems are protected from unauthorized access. Regular security checks and updates help to ensure the security of production systems.
How CorpIn supports companies with predictive maintenance
CorpIn offers companies comprehensive support in the implementation and optimization of predictive maintenance solutions. Our focus is on developing customized solutions that meet the specific requirements of each company and make maintenance processes more efficient.
One example of our support is the initial analysis of the data and system infrastructure. Before a predictive maintenance strategy is implemented, we carry out a comprehensive analysis of the existing data and systems. The aim is to ensure the quality and consistency of the data and identify potential weaknesses in the infrastructure. Companies benefit from a solid basis on which the AI models can work reliably.
Another area in which we support companies is the integration of sensor data and AI-supported data analysis. By implementing sensors that collect data in real time, companies can continuously monitor their machines and detect potential failures at an early stage. Our AI solutions analyze this data and provide valuable insights into the condition of the machines, which significantly improves the planning and efficiency of maintenance processes.
Finally, we attach particular importance to security and data protection measures. Predictive maintenance requires the use of IoT and cloud technologies, which is why data protection and cyber security are of central importance. We help companies implement the right security measures to protect their systems and meet the requirements of the industry.
Conclusion
Predictive maintenance is a powerful tool that helps companies to increase the reliability and efficiency of their production and save costs at the same time. Through the use of AI and IoT, predictive maintenance enables predictable and data-based maintenance that prevents unforeseen breakdowns. Companies that successfully implement predictive maintenance benefit from increased productivity and reduced downtime.
CorpIn offers tailor-made solutions that accompany companies on their path to predictive maintenance - from data analysis and the integration of sensor data to cyber security. Discover how we can help you optimize your maintenance processes and make your production facilities more efficient in the long term.
The content of this article may have been improved with the help of artificial intelligence. Therefore, we cannot guarantee that all information is complete and error-free.